AI Transforming In-Basket Management for Healthcare Providers
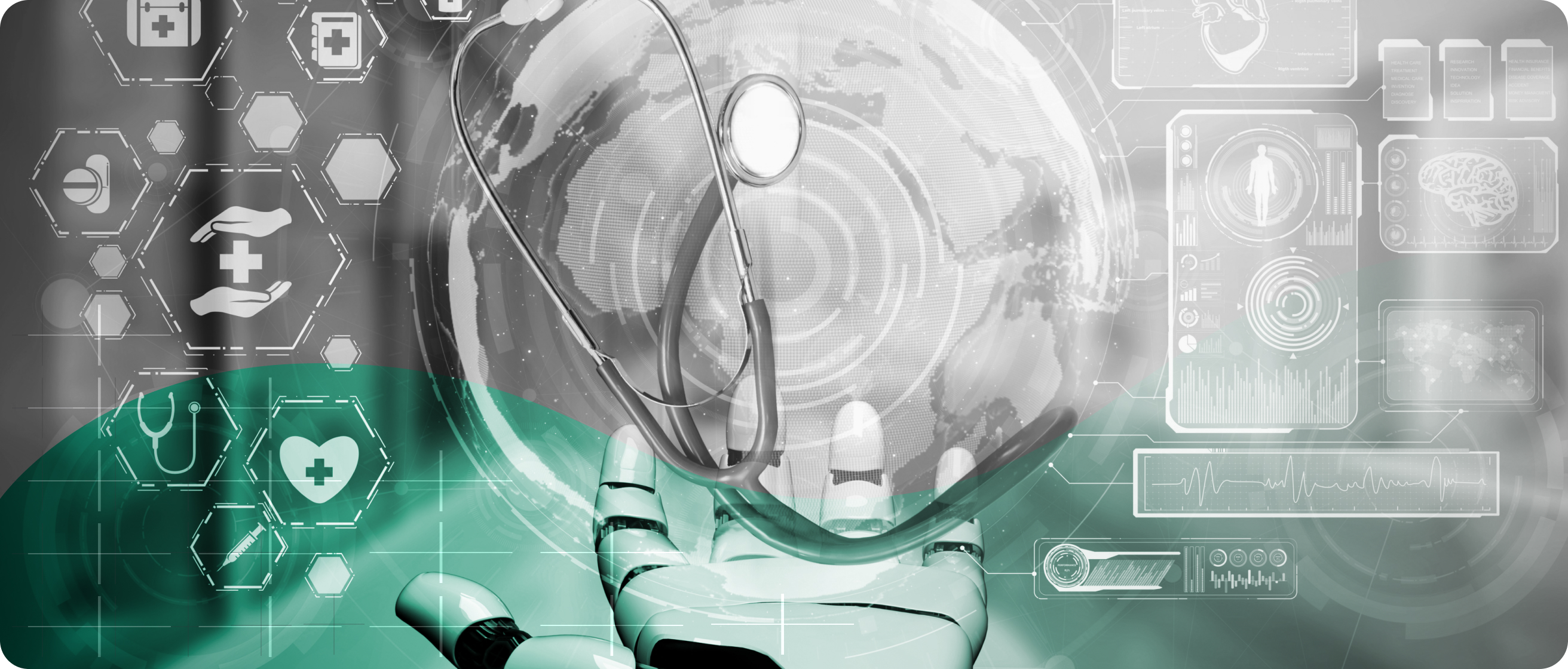
In-basket management, the healthcare industry’s equivalent of an email inbox, is a critical tool for communication between patients and providers. Through the in-basket, patients can send messages, request information, and ask questions, while healthcare providers can exchange messages with patients and among themselves. However, with the rise of digital tools such as patient portals (often referred to as online patient services), in-basket management has become more complex and burdensome for providers. The increasing volume of messages has added a new layer of administrative work for healthcare professionals. Enter AI, and specifically large language models, which offer the potential to streamline in-basket management and improve efficiency within healthcare systems.
The Role of Patient Portals in Expanding In-Basket Use
Patient portals have revolutionized patient-provider communication by giving patients more direct access to their healthcare teams. With these online platforms, patients can schedule appointments, view test results, request prescription refills, and most importantly, send messages to their providers. While patient portals have empowered patients and enhanced their involvement in their own care, they have also significantly increased the number of non-urgent and administrative inquiries that providers must address.
This influx of messages—ranging from simple questions about medications to more complex requests for health advice—places a significant load on healthcare providers who must manage these communications alongside their clinical duties. The result? A growing challenge for healthcare systems, where managing these in-baskets is time-consuming and can detract from other important tasks.
Defining the Problem Before Implementing AI
The first and most critical step in leveraging AI in healthcare is clearly defining the problem that needs to be solved. Too often, organizations adopt AI solutions without fully understanding the nuances of the issues they are trying to address. For instance, an AI model designed to predict hospital readmissions may not function as intended if it’s applied to a hospital with different patient demographics or healthcare protocols than the data used to train the model.
The key is to identify inefficiencies within the healthcare process that need to be addressed. Is the problem long patient wait times? Ineffective treatment plans? Or high rates of diagnostic errors? Understanding the exact nature of the inefficiency allows healthcare providers to determine whether AI is the right tool for addressing it. AI should be seen as a solution to a clearly defined problem, not a catch-all solution for every challenge in healthcare.
AI and Large Language Models: A Potential Solution
Artificial intelligence (AI), particularly in the form of large language models (LLMs), holds the potential to transform how healthcare providers manage their in-baskets. One of the key ways AI can assist is by helping to draft responses to patient messages. Currently, healthcare providers are tasked with responding to a wide variety of patient inquiries—many of which are routine or administrative in nature. AI-driven models can help by automatically drafting responses, which can then be reviewed and approved by a healthcare provider before being sent. This approach saves time and allows providers to focus on more complex patient care tasks, without sacrificing the personalized touch required in patient interactions.
Moreover, AI can assist with triaging messages within the in-basket. Not all patient messages carry the same urgency. For instance, a message from a patient experiencing new and worrisome symptoms requires more immediate attention than a routine request for medication refill information. AI can be programmed to categorize and prioritize these messages based on their urgency, helping providers reduce waiting times for patients in critical need and ensuring that the most important concerns are addressed promptly.
AI for Process Improvement and Pattern Recognition
Beyond streamlining communication, AI can help identify patterns within in-basket management that may not be immediately apparent to human providers. For instance, AI can analyze data to uncover trends related to message frequency, types of questions asked, or common patient concerns. This analysis can inform healthcare systems on how to adjust workflows to improve efficiency. Perhaps certain types of messages could be handled through automated responses, while others may require additional resources or adjustments in staffing to better manage the load.
By identifying these patterns, AI helps healthcare providers improve not only their in-basket management processes but also their overall approach to patient communication. This can lead to more efficient workflows, better resource allocation, and, ultimately, enhanced patient satisfaction.
The Future of In-Basket Management
In its current form, in-basket management can be inefficient, requiring significant manual effort from healthcare providers to respond to a growing number of patient messages. However, with the help of AI, the future of in-basket management could be far more streamlined and efficient. AI-driven systems can assist with drafting responses, triaging urgent messages, and identifying patterns that lead to better overall system design.
AI will not replace the human element in healthcare but rather enhance it, allowing healthcare providers to focus their attention where it’s needed most: providing high-quality care to their patients. By making the in-basket management process more efficient, AI can help reduce provider burnout, improve patient care, and ultimately, transform the healthcare experience for both providers and patients.
References
1. Holmgren, A. J., & Downing, N. L. (2022). The growing burden of inbox management. *JAMA Internal Medicine, 182*(5), 482-483. https://doi.org/10.1001/jamainternmed.2021.8314
2. Olson, C. A., Thomas, J. F., & Gill, M. J. (2019). Patient portals and health information exchange: Improving in-basket management in primary care. *American Journal of Managed Care, 25*(10), 497-502. https://doi.org/10.37765/ajmc.2019.43042
3. Ryu, S. (2020). Telemedicine: Opportunities and developments in member states. *World Health Organization, Global Observatory for eHealth series, 2*(1), 1-96. https://apps.who.int/iris/handle/10665/44497